Social Impact Analysis
Social impact analysis uses data analysis techniques and tools to understand the social and economic impact of a particular initiative, program, or policy. In this article, we explore how organizations can use impact analytics to measure the effectiveness of their interventions and identify ways to improve their impact.
Social impact analysis uses data analysis techniques and tools to measure the impact of a particular initiative, program, or policy on a specific outcome or set of outcomes. This could include analyzing crime rates, health outcomes, educational outcomes, employment outcomes, or other social and economic well-being measures. The goal of impact analytics is to understand the extent to which a particular intervention has had a positive or negative impact on the desired outcome and to identify ways to improve the effectiveness of the intervention.
Data analytics for social impact
Organizations increasingly realize the significance of analyzing their social impact in today's fast-paced world. However, this can often take time and effort, especially for organizations that cultivate a continuous learning culture.
Organizations or funders typically hire impact consultants or practitioners to navigate this complex landscape. These experts would then develop a logic model or theory of change, establish relevant metrics, and devise a plan for collecting data. They would then search for suitable surveys that align with their specific needs and expertise, whether online or offline. Finally, depending on the required dynamism, they would summarize the survey results using tools like PowerBI, Tableau, or other similar business intelligence platforms.
Unfortunately, this entire process is not only lengthy, but it also needs more repeatability for organizations. Each new program or project repeats the cycle with different funders. This inefficiency leads to a significant waste of resources and missed opportunities for organizations to learn and scale their programs or products effectively.
To overcome these challenges, organizations require a streamlined and scalable approach to analyzing their social impact. This involves leveraging impact model data analysis, which utilizes data to assess and evaluate the outcomes and effects of specific interventions, programs, or initiatives. By collecting, analyzing, and interpreting data, organizations can gain valuable insights into the effectiveness of their efforts and make data-driven decisions to optimize their social impact and achieve their desired goals.
The following sections will explore concrete best practices for designing a data model that analyzes social impact. But before we delve into these strategies, let's debunk some common misconceptions that hinder organizations from fully harnessing the potential of their data.
Social Impact Analysis Examples
Social impact analysis is a powerful tool that allows organizations to understand and evaluate the effects of their interventions, programs, or initiatives. By collecting and analyzing data, organizations can gain valuable insights into the impact and effectiveness of their projects and activities. Let's explore real-world examples of social impact analysis to understand its practical applications better.
Example 1: Education Program
Imagine an organization that runs an education program focused on improving literacy rates among underprivileged children. To assess the impact of their program, they collect data on various metrics such as reading levels, school attendance, and academic performance before and after the intervention. By analyzing this data, they can determine if their program has led to improvements in these areas. For instance, they might find that children who participated in the program showed significant growth in reading skills compared to those who did not. This information can then be used to refine and enhance the program, allocate resources effectively, and demonstrate the program's success to potential funders.

Example 2: Healthcare Initiative
Another example involves a healthcare organization implementing a community health initiative to reduce a specific disease's prevalence. They collect data on factors such as disease incidence, access to healthcare services, and patient outcomes. By analyzing this data, they can determine if their intervention has decreased disease rates, improved access to healthcare, and better health outcomes for the community. This information can guide future interventions, inform resource allocation decisions, and provide evidence of the initiative's impact on stakeholders and policymakers.

Example 3: Environmental Conservation Project
A third example focuses on an environmental conservation project working to protect a vulnerable ecosystem. The organization collects data on biodiversity, habitat quality, and community engagement. By analyzing this data, they can assess their conservation efforts' effectiveness, identify improvement areas, and measure the project's impact on the ecosystem and local communities. This information can guide future conservation strategies, inform policy decisions, and help secure funding for ongoing initiatives.
These examples illustrate how social impact analysis can provide valuable insights into the effectiveness of interventions, programs, and initiatives across various sectors. By collecting, analyzing, and interpreting data, organizations can make informed decisions, optimize their social impact, and work towards achieving their desired goals.

The table below provides a few technical challenges with social impact analysis.
Table: Different social impact analytics use cases
Impact Model Data Analytics
Impact model data analysis uses data to assess and evaluate the effects or outcomes of a specific intervention, program, or initiative. It involves collecting, analyzing, and interpreting data to understand the impact and effectiveness of a particular project or activity.
The analysis typically involves identifying key metrics or indicators that measure the desired outcomes of the intervention and then analyzing the data to determine the extent to which those outcomes have been achieved. This analysis can help organizations and stakeholders make informed decisions about resource allocation, program improvement, and strategic planning.
By utilizing impact model data analysis, organizations can gain insights into the effectiveness of their efforts and make data-driven decisions to optimize their social impact and achieve their desired goals.
This article will explore three concrete best practices for designing a data model that analyzes social impact. But first, let's debunk some common misconceptions to ensure a clear understanding.
Myths of Social Impact Data
In the modern age of data-driven decision-making, social organizations face unique challenges in deriving social impact from multiple data sources. Data plays a crucial role in understanding the effectiveness of programs, measuring impact, and optimizing resource allocation. However, misconceptions regarding data availability, quantity versus quality, and the need for experts to collect and analyze data often hinder organizations from fully harnessing the potential of their data.

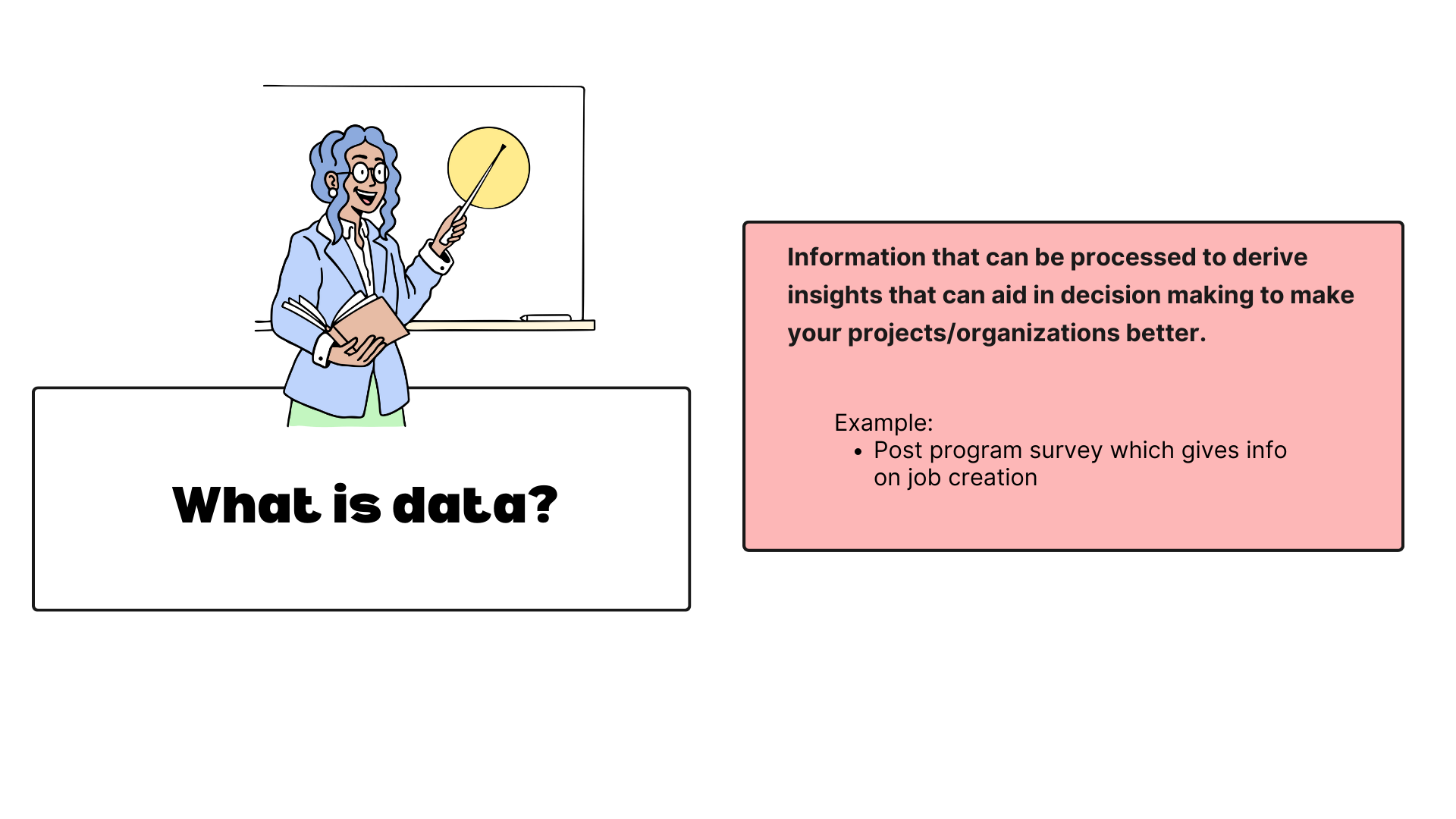
Myth #1: Some organizations don't have any data
One prevalent myth surrounding social organizations is the belief that some organizations lack data. In reality, most organizations have at least one or more data sources. For example, program registration data, surveys, and operational data are commonly collected by social organizations. Recognizing these existing data sources is crucial for organizations to unlock valuable insights and measure their social impact accurately.
To debunk the misconception that some organizations lack data, it is crucial to recognize the various data sources that organizations unknowingly collect. For instance, program registration data, surveys, and operational data are frequently gathered, even if organizations need to be aware of their potential. These data sources can be stored and managed using tools like Excel, Google Sheets, or more advanced platforms like Salesforce or custom software. Organizations can uncover valuable insights and drive meaningful impact by harnessing existing data sources.

Myth #2: The more data we have, the better
Another misconception is that the more data an organization has, the better. However, data quantity does not necessarily equate to data quality. It is essential to focus on the relevance and accuracy of the data rather than sheer volume. Organizations must ensure that the data they collect is aligned with their goals and objectives, enabling them to make informed decisions and measure their social impact effectively. Strategies for managing and analyzing large volumes of data can also help organizations derive actionable insights.
While data quantity may seem significant, the relevance and accuracy of the data genuinely matter. Collecting and analyzing relevant data aligned with an organization's objectives enables more accurate social impact measurement. Organizations should focus on gathering data that quantifies outcomes rather than subjective goals, making impact measurement less reliant on personal interpretation. By prioritizing data relevance, organizations can enhance the effectiveness of their programs and initiatives.
Myth #3: Organizations need experts to collect and analyze data
Many organizations believe collecting and analyzing data requires specialized skills or dedicated experts. However, empowering organizations to collect and analyze their data can significantly enhance their ability to measure social impact. Various tools and resources are available to simplify data collection and analysis processes, making them accessible to organizations of all sizes and capacities. Organizations can gain valuable insights and drive positive change by taking ownership of data collection and analysis.
Organizations often believe data collection and analysis require expert knowledge or specialized skills. However, various tools and resources exist to empower organizations to collect and analyze their data. Platforms like SurveyMonkey, Google Forms, or custom-developed tools can simplify the data collection, while tools like Monday or Excel can aid data analysis. By demystifying data collection and analysis, organizations can harness the power of data without the need for extensive expertise.
Debunking the Myth
Contrary to popular belief, organizations can use more expertise or dedicated experts to collect and analyze data. The key is simplifying data collection and analysis processes, making them accessible to the organization's more comprehensive users.
Tools and software platforms, such as customer relationship management (CRM) systems, data warehouses, and analytics tools, provide user-friendly interfaces that enable organizations to collect, manage, and analyze data effectively. These tools often offer data cleaning, deduplication, and integration features, simplifying the data management process.
By leveraging these tools and platforms, organizations can empower their team members to take ownership of data collection and analysis. This promotes a culture of data-driven decision-making throughout the organization and reduces reliance on external experts.
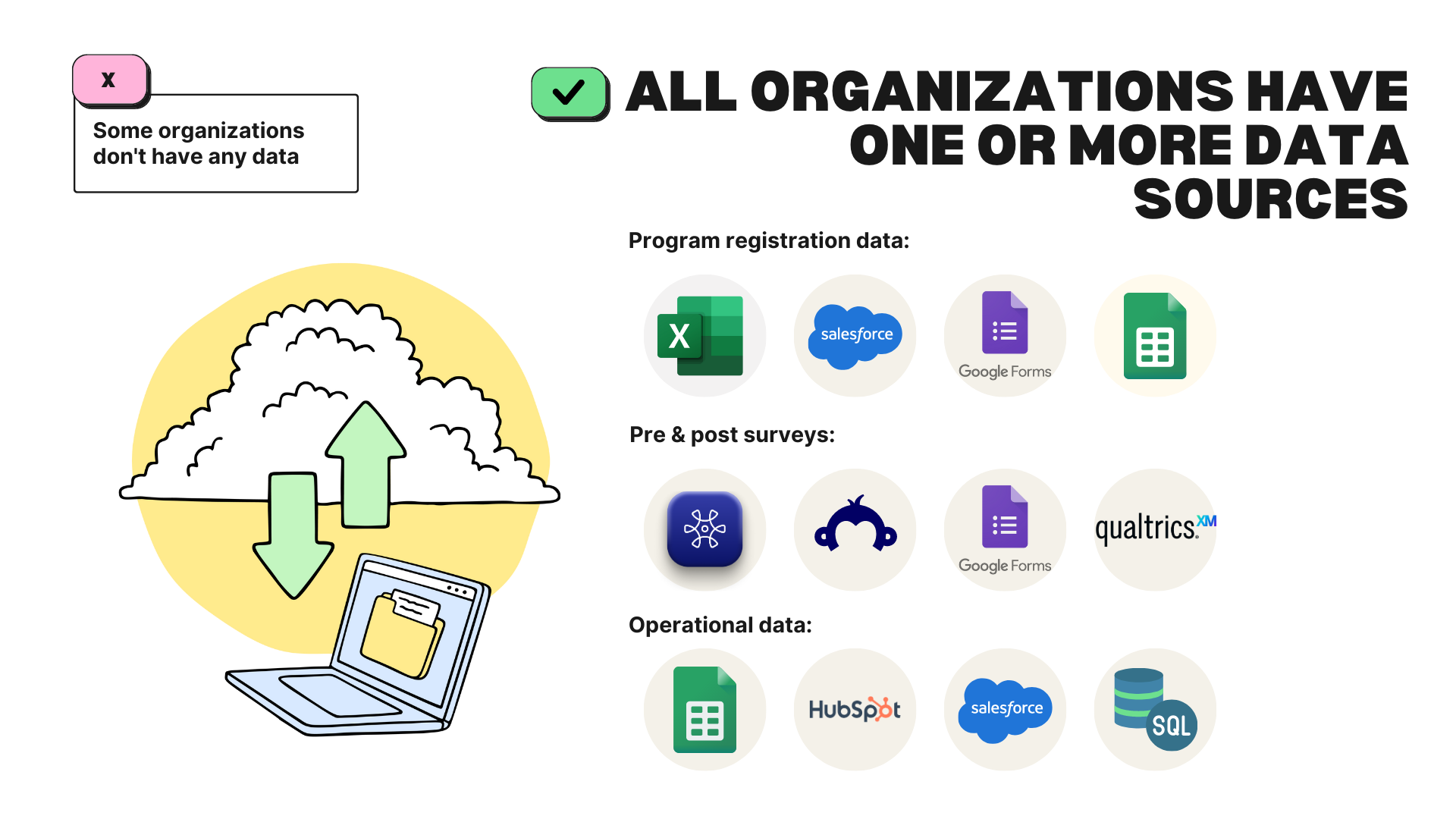
The Importance of Data Relevance and Simplifying Data Collection and Analysis
The earlier section delved into the misconception that having more data is always better. However, it's crucial to balance gathering large amounts of data and ensuring its relevance to the organization's goals. While there are situations where collecting more data is justified, it is crucial to be intentional about what we want to measure. The following section will explore the intricacies of organizations' common obstacles during their data collection journey and provide practical and effective solutions to overcome them.
The upcoming section will offer a comprehensive, step-by-step guide on effectively designing and implementing social impact data management strategies. Let's concentrate on three critical areas integral to this process to gain a deeper understanding.
- Quality of Data
- Intentional Data
- Integratable Data

Quality of Data
One standard problem organizations encounter is the need for more uniquely identifiable information. It is essential to have a unique identifier for each stakeholder or participant to connect data from different sources accurately. This identifier doesn't have to be personal information like names or email addresses; it can be a random identification code. A unique ID helps triangulate data from various sources and ensures accurate analysis.
%252520Obstacles%252520to%252520deriving%252520impact%252520from%252520multiple%252520data%252520sources.png)
Typos and inconsistent data entry can cause problems when analyzing data. Different individuals may enter information in various ways, leading to data discrepancies. It is essential to address this issue by establishing data entry guidelines and ensuring consistency in recording information. Organizations can prevent data quality issues and facilitate meaningful analysis by standardizing data entry.
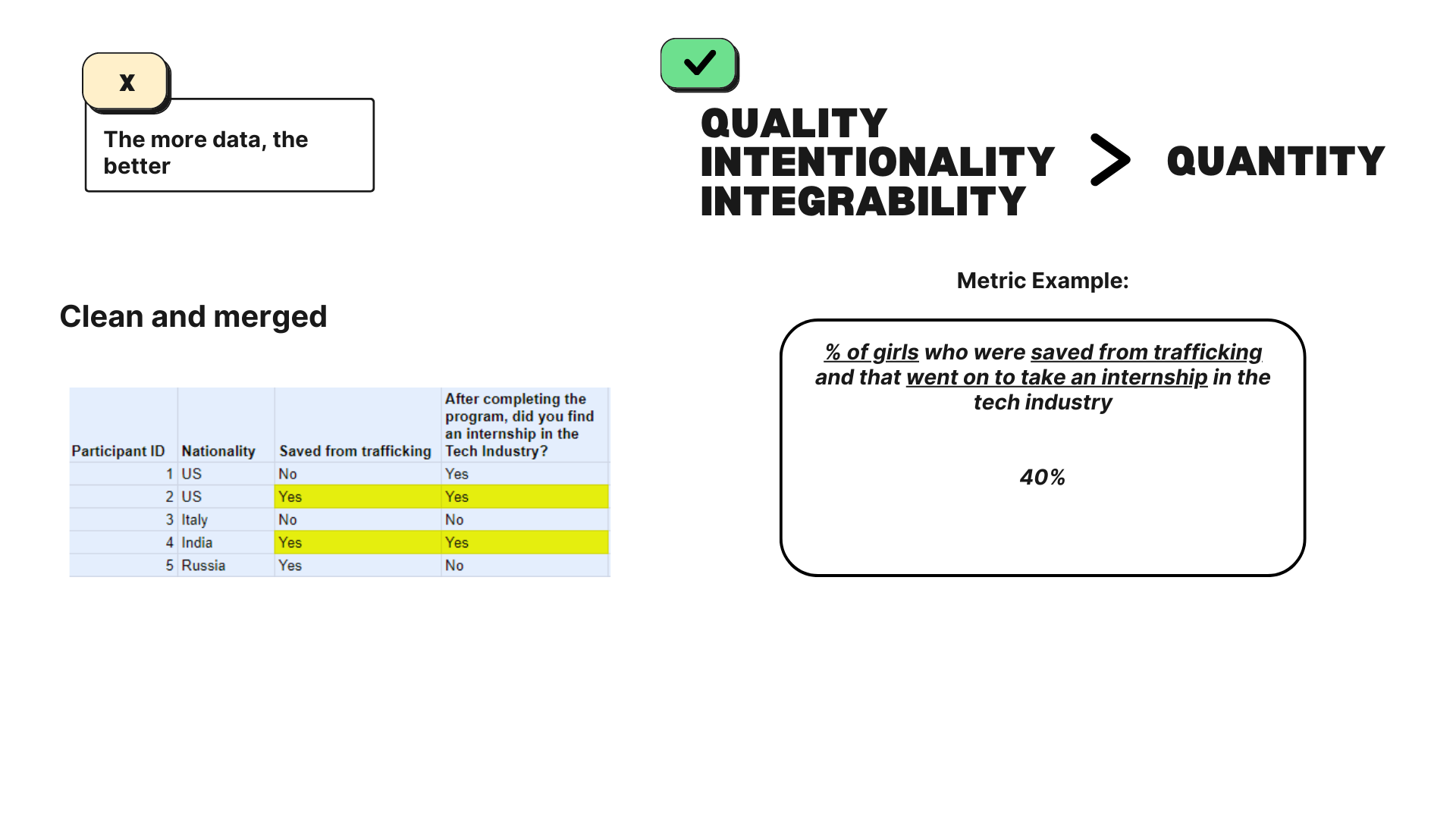
Duplicate records occur when the same stakeholder or participant is entered multiple times in the data collection system. This can happen due to manual errors or system glitches. Duplicate records can distort the accuracy of analysis results, leading to misleading insights. Implementing data deduplication processes and regularly auditing data can help organizations identify and remove duplicate records, ensuring the integrity of their data.
Another challenge organizations face is storing data in a format that could lend itself better to analysis. Data should be stored in a structured manner allowing easy retrieval and analysis. Using data management systems or data warehouses can help organizations organize their data effectively, enabling them to run accurate and insightful analyses.
Intentional Data Collection
Intentional data collection is crucial to obtaining valuable insights and ensuring data quality. It goes beyond simply having access to advanced tools or investing significant money. Instead, organizations must establish consistent and effective data collection practices aligned with the nonprofit logic model. By doing so, they can focus on the specific questions and metrics that align with their goals, avoiding data overload and maintaining a clear focus on key metrics.
This intentional approach allows organizations to streamline their data collection efforts by asking targeted questions and honing in on the most relevant data points. As a result, they can ensure higher response rates and generate meaningful insights that drive positive change.
For example, if an organization aims to enhance stakeholder outcomes, it can collect stakeholder profiles and baseline information in platforms like Salesforce, where stakeholders are likely to be present. Alternatively, they can utilize well-designed surveys like Sopact to periodically assess their progress in improving stakeholder primary outcomes. Organizations can optimize their data management practices by emphasizing the importance of intentional data collection and paving the way for data-driven decision-making.

CRM systems like Salesforce and Zoho offer robust data management and analysis features. They make extracting data from the system relatively easy, enabling organizations to access and retrieve the necessary information. However, the challenge lies in the process of putting data into CRM.
To collect good data, organizations must have well-defined and consistent practices that guide the data collection process. Here are some key considerations:
- Standardized Data Entry: Establishing guidelines for data entry ensures consistency across the organization. Organizations can avoid common pitfalls such as typos, inconsistent data, and incomplete information by defining specific formats, units, and conventions. Consistent data entry practices contribute to the accuracy and reliability of the collected data.
- Clear Data Fields: Clearly defining each data field's purpose and required information helps streamline the data collection. It ensures that the right data points are captured and eliminates unnecessary or redundant information. By focusing on relevant data fields, organizations can optimize their data collection efforts and enhance the quality of the collected data.
- Regular Data Audits: Regular audits of the collected data are essential to identify and rectify discrepancies or errors. By reviewing the data periodically, organizations can maintain data integrity and identify areas that require improvement. Regular data audits help ensure that the data collected remains accurate, reliable, and up to date.
- Data Integration: Integrating data from various sources into a centralized system, such as a data warehouse, enhances data management and analysis capabilities. It allows organizations to connect different data streams, eliminate data silos, and gain a comprehensive view of their operations. Data integration facilitates cross-referencing and cross-analysis, enabling organizations to derive deeper insights and make more informed decisions.
- Training and Education: Providing proper training and education to employees involved in data collection is crucial. It ensures that individuals understand the importance of data quality, adhere to data collection practices, and utilize the available tools effectively. Investing in employee training promotes a culture of data-driven decision-making and empowers the workforce to contribute to the organization's data-collection efforts.
By implementing these practices, organizations can collect good data consistently and unlock valuable insights. I want you to know that suitable data collection practices should be embraced throughout the organization, not limited to specific departments or individuals, is essential. Every organization member should understand their role in maintaining data quality and strive for excellence in data collection.
Remember, the goal is not merely to collect data but to collect the correct data with intention. With intentional data collection practices in place, organizations can harness the full potential of their data, gain actionable insights, and make well-informed decisions that drive positive outcomes.
Integratable Data
Data integration and cleaning are crucial steps in the data analysis process. If data from different sources remains connected, analysis becomes manageable and prone to errors. Connecting data sources through tools like data warehouses allows organizations to integrate data seamlessly and perform comprehensive analyses. It also helps automate data cleaning processes, reducing the chances of human error and improving data accuracy.
Conclusion
This article explored common data collection problems and debunked the myth that organizations need experts to collect and analyze data. Organizations can derive valuable insights and make informed decisions by focusing on data relevance, addressing data quality issues, and simplifying data collection and analysis processes.
It is essential to collect intentional data, asking the right questions that align with the organization's goals. By standardizing data entry, implementing data deduplication processes, and leveraging tools for data integration and cleaning, organizations can ensure the accuracy and reliability of their data.
By simplifying data collection and analysis processes and empowering internal teams, organizations can harness the power of data to drive impact and create positive change.
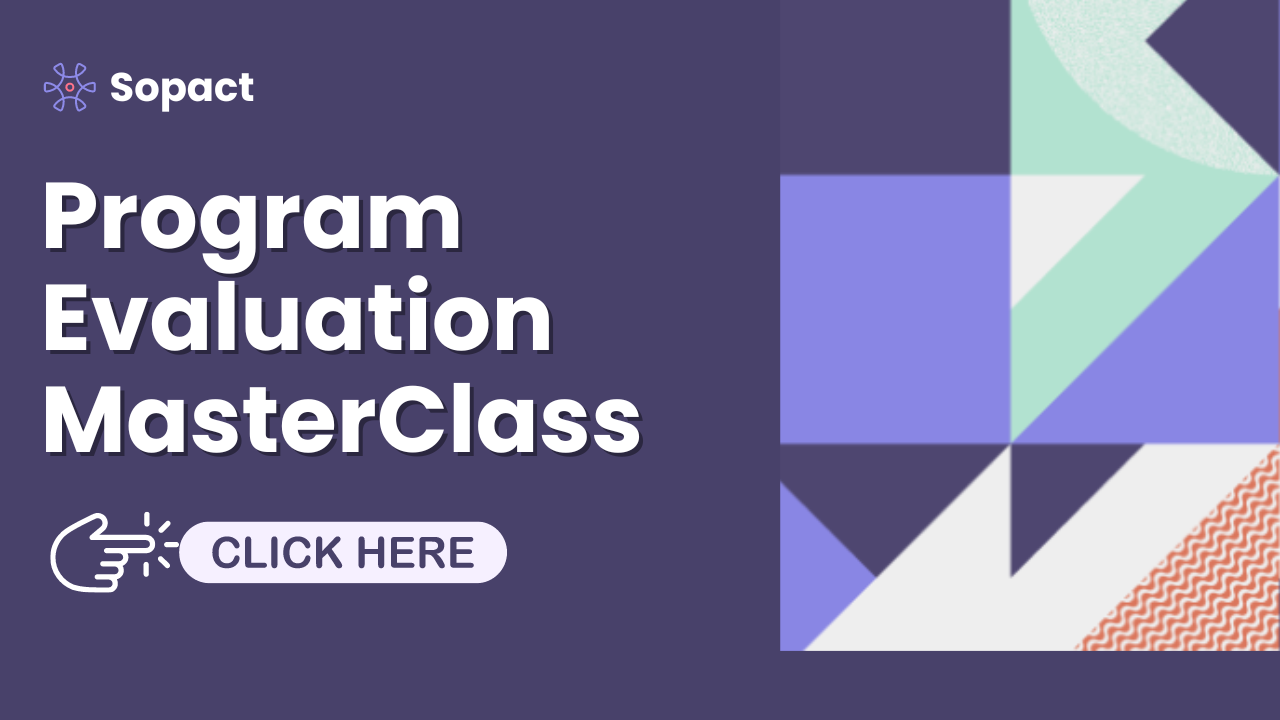